Just What the Doctor Ordered

Human beings are some of the most complex systems in the world, and responses to illness, disease, and impairments manifest in countless different ways. When it comes to making sure that your system stays up and running, healthcare professionals typically have their own deep well of knowledge—but the addition of artificial intelligence tools offers unprecedented support from millions of data points, global expert opinions, and the objective conclusions that only machine learning can provide. College of Engineering researchers are putting artificial intelligence to work conducting research in a wide range of areas in the healthcare industry.
This article originally appeared in Engineering @ Northeastern magazine, Spring 2021.
Coming to an understanding
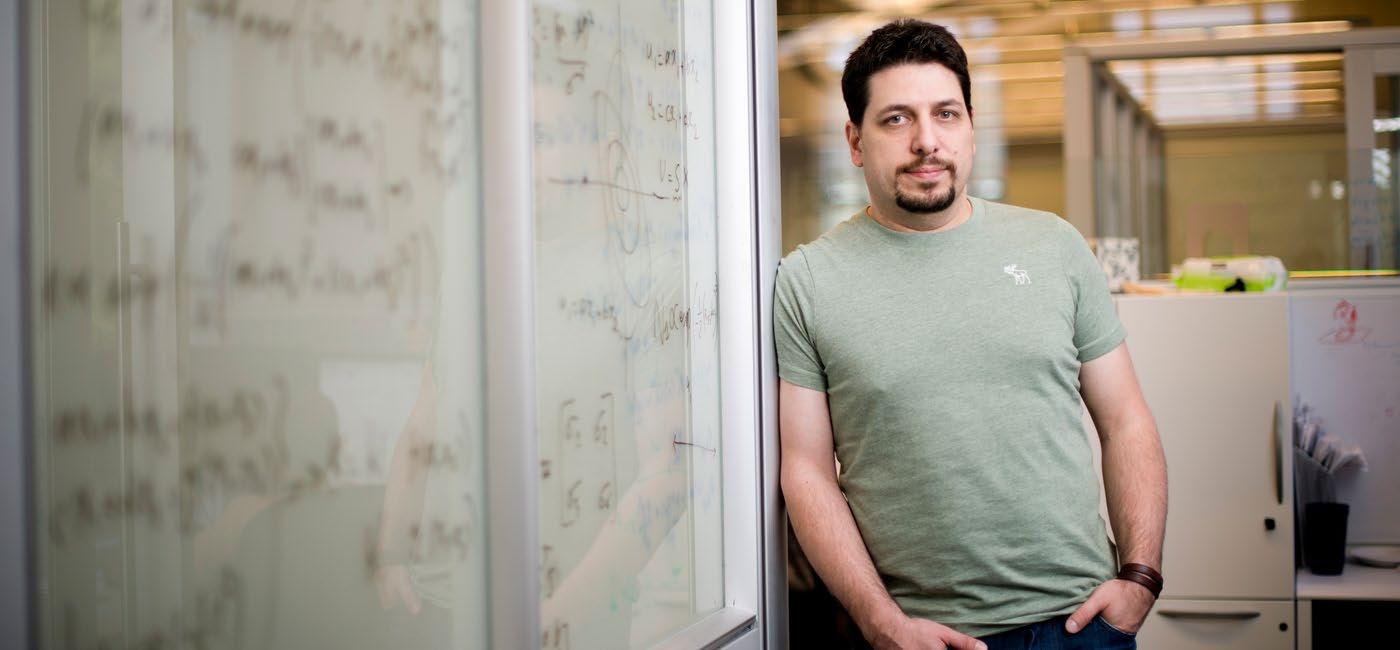
Deniz Erdogmus, professor, electrical and computer engineering; photo by Northeastern University
Professor Deniz Erdogmus, electrical and computer engineering, is working with experts at Oregon Health & Science University (OHSU) on assisted communication devices for people with speech and/or physical impairments.
The project started in 2009 as a brain/computer interface that allowed users to express themselves using an RSVP Keyboard™, which works by identifying changes in the brain’s electrical activity when viewing flashing letters on a computer screen. Erdogmus and his team are combining data from the user’s brain signals along with context-specific language models from their conversation partner and other environmental factors.
“We just got our third renewal on our National Institutes of Health grant,” says Erdogmus. “In this next five-year phase, we’ll be looking at data from eye tracking, muscle activity through electromyography (EMG), and button presses as well. Because each user is different in terms of their neurological conditions and body control, we want to be able to give them options on the best way to use their communication device.”
A significant advantage of this multimodal communication system is for people with progressive diseases like amyotrophic lateral sclerosis (ALS), who might have some muscular capabilities earlier in their disease that might disappear with time—but because the device has learned their individual signals, it can still help them communicate with ease.
From the first days of life …
Another OHSU collaboration sees Erdogmus partnering with ophthalmologists on an NIH-funded grant to better identify and treat retinal disease in premature infants.
“When babies are born prematurely, there is frequently a concern about retinopathy of prematurity (ROP), a disease in which the retina is not fully or properly formed yet,” says Erdogmus. “If detected and assessed properly, ROP can be treated to prevent vision loss, but outside of urban areas the technology for identifying it isn’t as widely available.”
With a network of healthcare providers in rural North American and underdeveloped countries such as India and Nepal, Erdogmus and his team are working with retinal images of preemies from specialized cameras. Using a neural network to identify and classify problem signs in the images, the team seeks to create a mobile imaging platform that can be used anywhere to get a better, faster diagnosis of ROP.
The U.S. Food & Drug Administration has granted the team’s mobile device technology a Breakthrough Devices classification.

Sarah Ostadabbas, assistant professor, electrical and computer engineering
In another project on early development, Assistant Professor Sarah Ostadabbas, electrical and computer engineering, is working with researchers across Northeastern and at the University of Maine using artificial intelligence to examine the interplay between pacifier use and sudden infant death syndrome (SIDS).
“Disruption of motor development in infancy is a risk indicator for a host of developmental delays and disabilities and has a cascading effect on multiple domains: social, cognitive, memory, and both verbal and non-verbal communication,” says Ostadabbas. “Despite the clear advantages of early screening, only 29% of children receive some form of developmental testing. Automation of home-based screening tests can increase the percentage of children who are looked at for early delays and atypical development.”
While many U.S. families have baby monitors that supply a wealth of visual data about babies’ behaviors, physicians don’t have the time to observe them for clues. That’s where automation comes into play: Ostadabbas is using her expertise in computer vision and advanced AI to create algorithms that can search for and identify sleep posies, facial expressions, and pacifier use behavior poses that can potentially signify developmental delays in infants.
These algorithms, however, are very data-hungry, and there are security and privacy issues that come along with using images and video of infants. In order to work around this “small data” problem, Ostadabbas has developed data efficient machine learning approaches to enable advanced AI algorithms for this application.
To mitigate the data limitation issue and towards developing a robust infant behavior estimation/tracking system, Ostadabbas’ technique has a framework that is bootstrapped on both transfer learning and synthetic data augmentation approaches. First, it makes use of an initial pose estimation model trained on the abundant adult pose data, and then finetunes the model on an augmented dataset consisting of a small amount of real infant poses and a series of synthetic infant videos using simulated avatars.
Because nothing simulated is exactly like real life, Ostadabbas has also developed a context invariant representation learning algorithm that helps narrow the data distribution gap between the infant avatars and real infants’ appearances.
“The specific application here is on a project about infant behavior monitoring, but the concept of small data problems runs throughout many domains, including much of healthcare and the military,” says Ostadabbas. “Besides AI models that we create, we are also making several publicly available datasets in small data domains; everyone needs more data, and we want to help advance the science.”
The National Science Foundation has funded the collection of some of Ostadabbas’ datasets related to sleep pose estimation studies.
… to the Golden Years
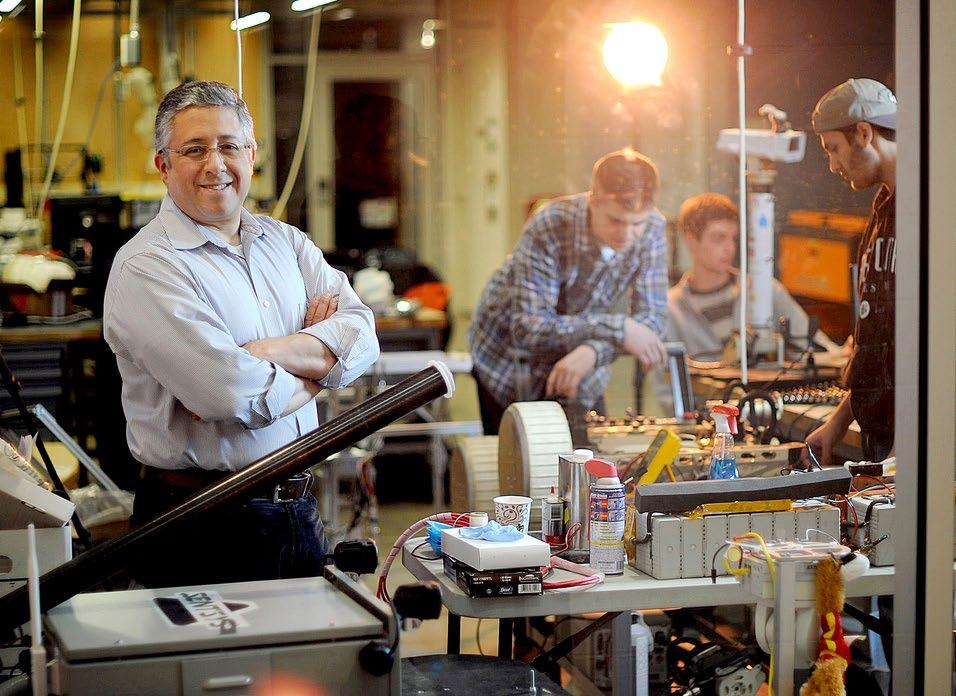
Taskin Padir, associate professor, electrical and computer engineering; photo by Mathew Modoono
Associate Professor Taskin Padir, electrical and computer engineering, has embarked on a new collaboration with researchers from Japan on developing technology for healthy aging and longevity in the home in adult populations. In both countries, older adults are living longer and more active lives, which will necessitate new technology to help them stay healthy and independent.
“Both qualitative and quantitative data showed us that the global pandemic had dire consequences on older populations,” says Padir. “We need to provide meaningful technology to keep them engaged and empowered, but that also has the capability to care for them.”
In a time when taking a taxi or a Lyft to get to the grocery store isn’t an option, Padir and his collaborators are seeking use-inspired and data-driven approaches to provide safe mobility between home and everyday destinations and can be used by family to check up on elderly relatives.
“We are really going back to the drawing board with what this future mobility device could look like,” explains Padir. “It’s not a wheelchair or a Segway, but what is in between? Is it foldable or shape-changing so I can both sit or stand on it? Is it indoor/outdoor? Can it help me with my cooking or cleaning? The possibilities are endless.”
From point A to point B
Another of Padir’s projects seeks to create an assisted mobility system for individuals with disabilities. His research focuses on bridging the technological gap between getting the robot to understand human intent, preferences, and expectations and accomplishing the correlating tasks with the machine’s capabilities.
“For more than a decade we’ve been looking at utilizing robots for health applications—not only in homes and hospitals, but also in common spaces,” says Padir. “This can’t be achieved just by algorithms, so we’re relying more on human feedback and giving a person adaptive use of the technology; not one solution will fix all needs, and not the same solution will fit the same person all the time.”
Padir’s vision for his assisted mobility technology ranges from a traditional wheelchair with a joystick to a self-driving wheelchair that takes in some level of human input, such as gestures or brain signals, to make sense out of the user’s intent. Padir and his team have gotten feedback from more than 250 users so far to understand their day-to-day activities and challenges, such as navigating a subway station during rush hour, or traveling on the sidewalk without running into people.
“We’ve often heard that the first mile and the last mile are the hardest for people with disabilities trying to make their way from place to place,” says Padir. “Once you’re on the subway, or the train, or the airplane, things are relatively easy—but the challenge is getting there and back.”
Padir’s human-robot interaction project has been through some in-home environment trials, but he now seeks to take the show on the road. His vision is to help a resident of The Boston Home, an assisted living facility in Dorchester, Massachusetts, to the Boston Symphony Orchestra and back using MBTA transit and the independent mobility provided by the robotic device.
Offering more comprehensive care
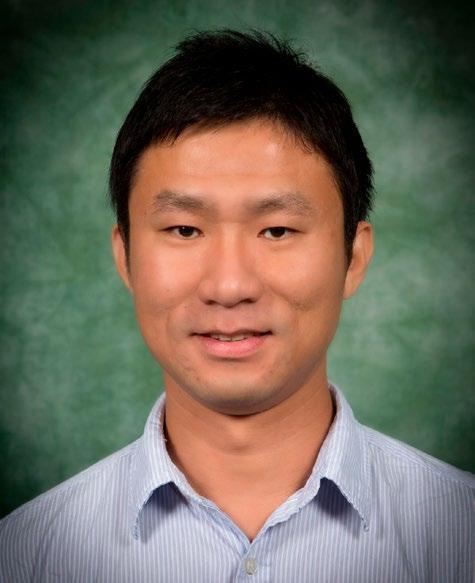
Chun-An (Joe) Chou, assistant professor, mechanical and industrial engineering
Assistant Professor Chun-An (Joe) Chou, mechanical and industrial engineering, is using machine learning techniques to help provide objective feedback to medical professionals on sensory or motor impairments in patients after strokes to improve rehabilitation.
In collaboration with the The Stroke Center at Tufts Medical Center, Chou— with Professor Yingzi Lin and Associate Clinical Professor Sheng-Che Yen—has developed a virtual reality driving simulator to test patients’ response behaviors and timing.
Chou’s research focuses on people about 2–6 months after a mild stroke who have moderate impairments. Their hypothesis is that by better identifying their issues, they can help people return to normal life activities more quickly.
“By using automation, we can provide personalized guidance for the medical provider in identifying real-time responses,” says Chou. “From a delay in noticing a pedestrian crossing the street to not seeing a stop sign, the patient’s cognitive responses can be categorized using artificial intelligence to offer providers a window into their impairments and recovery.”
This AI-based assessment tool can be generalized to other diseases/disorders causing relevant impairments or disabilities.
Chou has been awarded a Tufts CTSI Pilot Studies Program grant as the principal investigator of this project.
Monitoring patient behavior
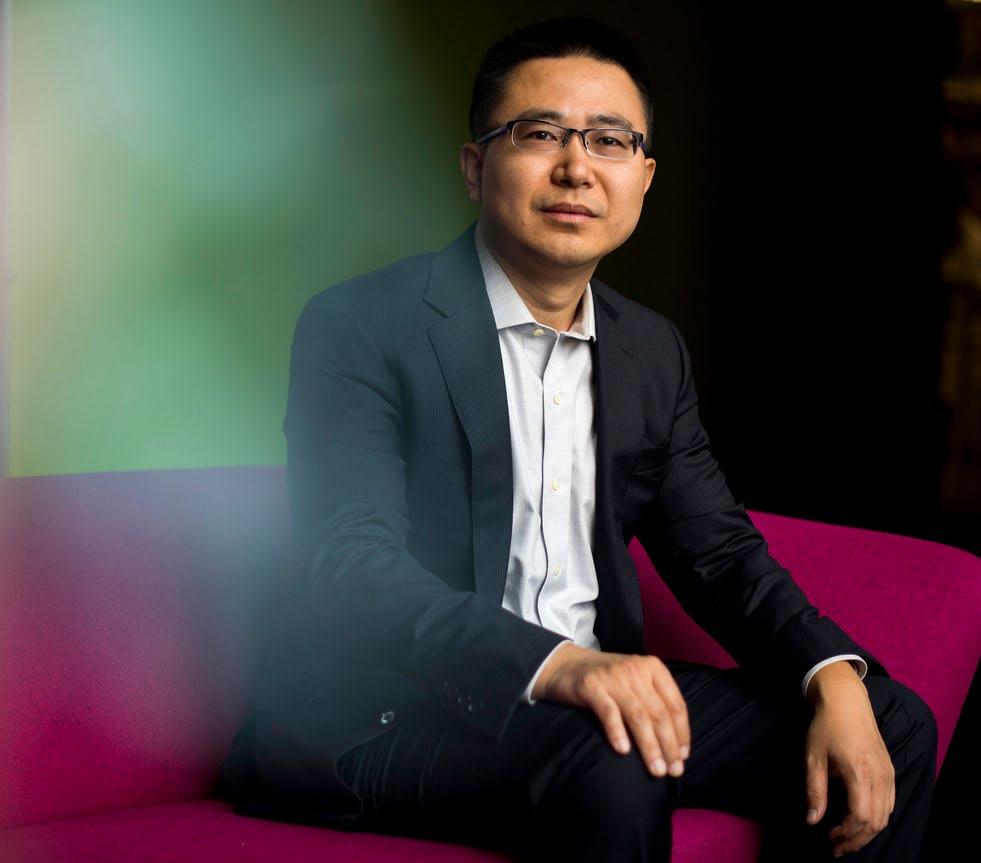
Raymond Fu, professor, electrical and computer engineering; photo by Mathew Modoono
Another Northeastern researcher seeking to augment patient care is Professor Raymond Fu, electrical and computer engineering. He is putting his experience using low-cost and portable sensors to work in digital healthcare by monitoring patient behavior during rehabilitation and exercise to increase effectiveness and decrease injury.
Using artificial intelligence to analyze data from sources like muscle sensors, 3D motion capture sensors, camera sensors, and more, Fu seeks to teach the AI system to generate alerts about anomalies or suggest additional services to facilitate researchers, therapists, and doctors in their practice.
“In physical therapy or rehab, patients want to follow their therapist’s practice on their own,” says Fu. “By teaching AI to perform recognition, classification, tracking, and analysis—all remotely, quickly, and highly efficiently—we can help health practitioners offer instantaneous feedback and support.”
Fu has been working on this research since 2012 and advancing technology has allowed him to utilize more advanced tools, including camera data from patients’ phones, laptops, or tablets; more lightweight sensor technology; and the surge of interest in remote health applications—especially in the wake of a global pandemic.
“The evolution of technology in the human activity recognition field over the last decade has gone from expensive to cheap, and from relatively weak to incredibly powerful,” says Fu. “It allows the integration of neural networks and machine learning to be more feasible and run in real-time, and users can be very decentralized from the cloud. So much research was just sitting in the lab and not being applied because the speed and the infrastructure just weren’t there to support it—but now they are.”
Protecting life-saving medicines

Wei Xie, assistant professor, mechanical and industrial engineering
The biopharma industry is growing rapidly and is increasingly able to cure severe health conditions, such as many forms of cancer and adult blindness. However, drug shortages have occurred at unprecedented rates over the past decade, especially during the COVID-19 pandemic.
Assistant Professor Wei Xie, mechanical and industrial engineering, is working with partners in both academia and industry to develop risk-based interpretable artificial intelligence to accelerate end-to-end biopharmaceutical manufacturing innovations and improve production capabilities.
Protein drug substances are manufactured in living cells, whose biological processes are complex and have highly variable outputs depending on complex dynamic interactions of many factors, such as intracellular gene expression, cellular metabolic regulatory networks, and critical process parameters. In addition, the biomanufacturing production process usually includes multiple steps, from cell culture to purification to formulation. As new biotherapeutics (e.g., cell and gene therapies) become more and more `personalized,” biomanufacturing requires more advanced manufacturing protocols. Thus, the productivity and drug quality are impacted by the complex dynamic interactions of hundreds of factors.
“If anything along the biopharma manufacturing isn’t exactly right, the structure of the protein can become different, which directly impacts drug functions and safety,” says Xie. Further, the analytical testing time required by biopharmaceuticals of complex molecular structure is lengthy, and the process observations are relatively limited.
To maximize productivity and ensure drug quality as measured by FDA required standards, Xie’s research team is employing risk management, process optimization, and interpretable AI. Through collaborating with Physical Sciences Inc. and UMass Lowell, we can monitor in real-time the critical variables, from the metabolic health of each individual cell to the entire manufacturing process.
“Our risk-based interpretable AI approach can advance the deep scientific understanding of biological/ physical/chemical mechanisms for protein synthesis at molecular, cellular, and system levels, support the real-time release, and accelerate design and control of biopharmaceutical manufacturing processes,” says Xie. “This study can proactively identify and eliminate bottlenecks and anomalies, accelerate production process development, and improve production capabilities and sustainability.”
Xie has recently completed a research proposal for the National Institute for Innovation in Manufacturing Biopharmaceuticals (NIIMBL) with Northeastern’s Biopharmaceutical Analysis Training Lab (BATL), MIT, Sartorius, Genentech, Centuria, Janssen, and Merck that seeks to bring this AI technology to market and support the biomanufacturing workforce innovations.
“This advanced sensor technology combining with interpretable AI can ensure that we produce high-quality bio-drugs and vaccines quickly and safely,” says Xie.
Understanding Alzheimer’s progression
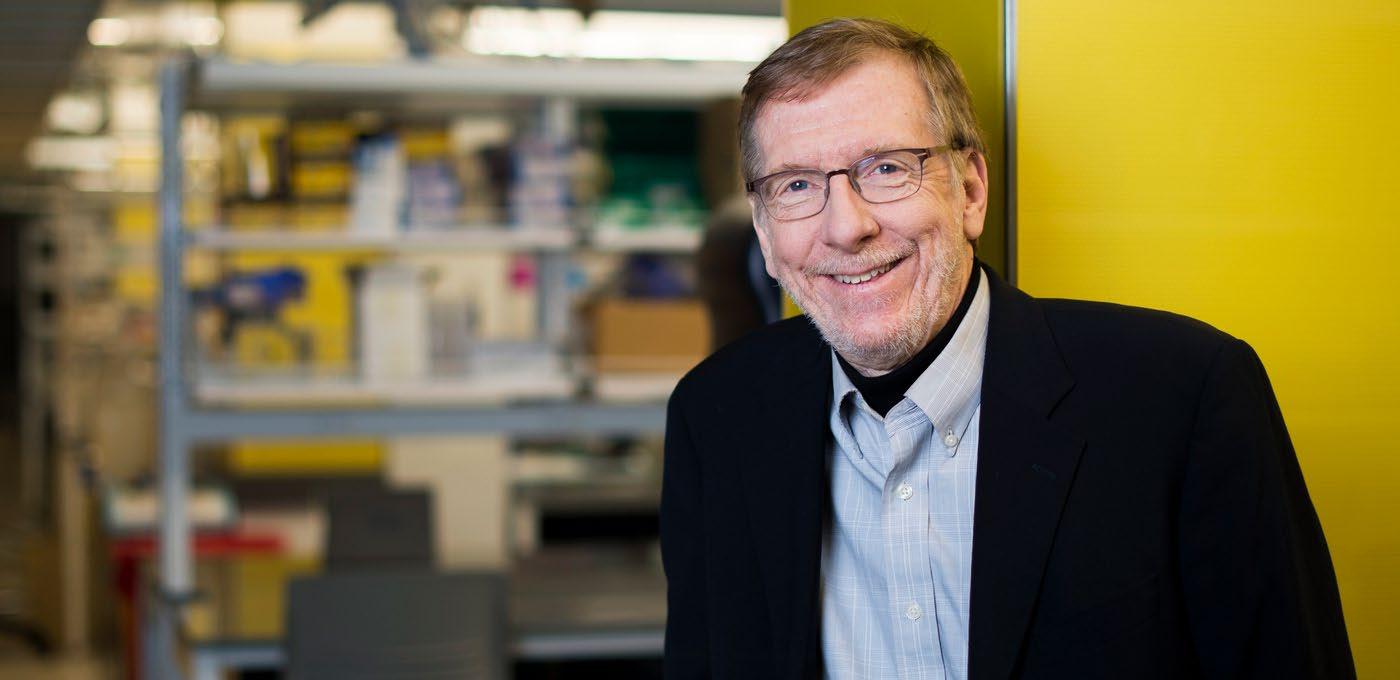
Lee Makowski, professor and chair, bioengineering; photo by Adam Glanzman
Professor and Chair Lee Makowski, bioengineering, aims to understand the molecular basis for the progression of Alzheimer’s disease by shooting X-rays at thin histological sections of human brain tissue to collect and examine data on the structure of lesions.
“Alzheimer’s is perplexing because it works so slowly, over a period of years, even decades,” says Makowski. “An even bigger issue is that the molecular lesions (known as amyloid plaques) don’t correlate well to the disease’s symptomology: Someone who has deep dementia may not have many lesions in their brain, and someone with many lesions may not suffer dementia. We’re trying to find out why that is.”
One of the theories Makowski and his team are investigating is that there are different types of amyloid plaques, with some being neurotoxic and others benign. Using artificial intelligence algorithms to evaluate the histological images, they hope to learn how to tell the difference at the molecular level so that doctors can eventually focus therapies on the lesions that are most dangerous.
Interestingly, some of the algorithms that Makowski is using have been around for a long time—but it’s only because of the advent of higher computing power and data storage in recent years that make them more useful.
“The data we’re collecting is modest by Big Data standards, but 10 years ago couldn’t have afforded the disk space to store it—now you can purchase enough storage at Best Buy to last us years,” says Makowski. “The availability of data storage and more powerful computational platforms have been game-changers in terms of our ability to use artificial intelligence to investigate these issues. The next five years in bioengineering are going to be incredibly productive because of new resources.”
Predicting molecular mechanisms of disease
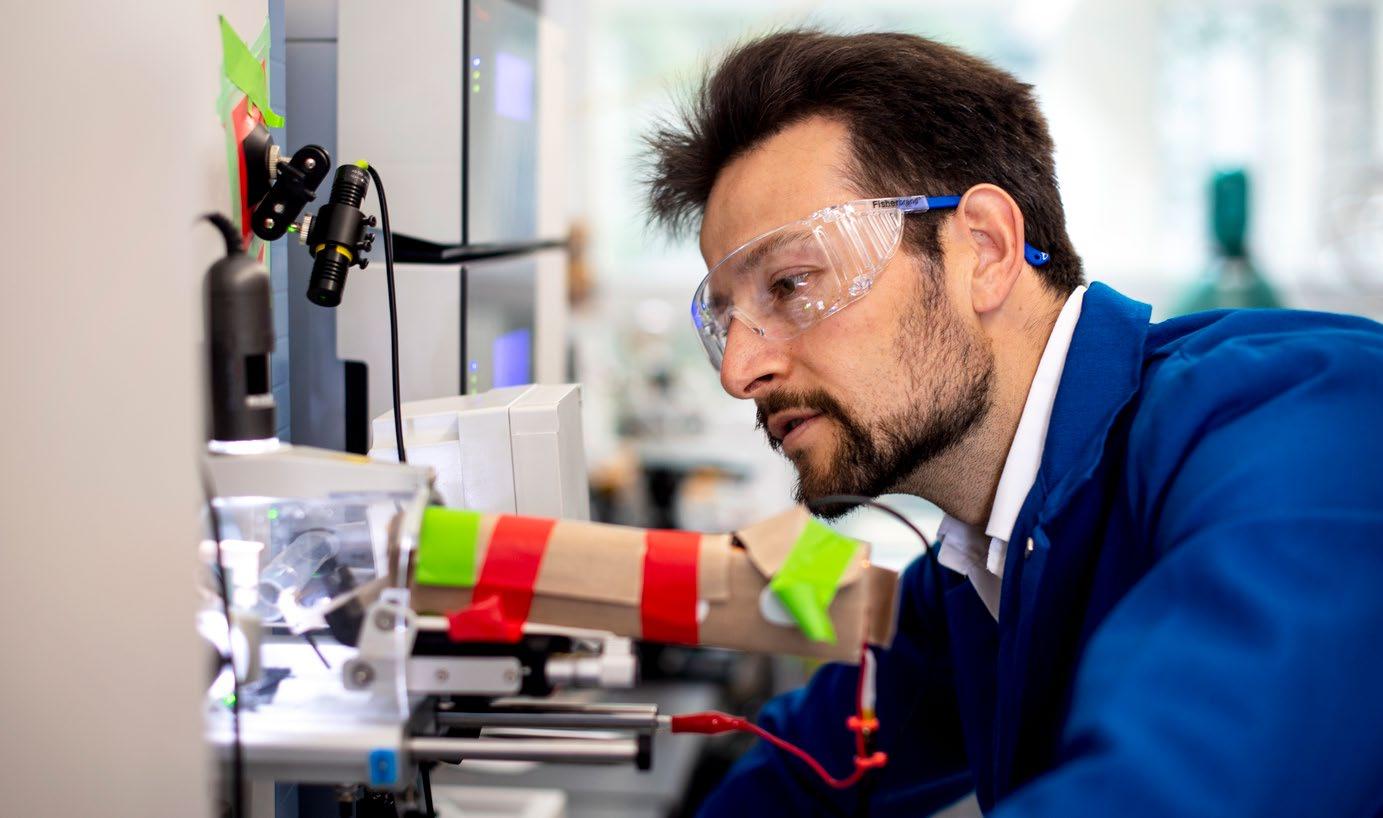
Nikolai Slavov, assistant professor, bioengineering; photo by Ruby Wallau
Assistant Professor Nikolai Slavov, bioengineering, is using AI and machine learning to predict molecular mechanisms of disease through protein analysis of thousands of single cells. Slavov is currently interested in examining how diseases such as cancers and autoimmune disorders interact with human macrophages, a type of immune cells.
“When macrophages come in contact with cancer cells, they can either attract and kill them or protect them,” explains Slavov. “We want to determine what are the molecular variations that make macrophages act differently so that we can potentially convert one type to another to increase the body’s effectiveness at fighting cancer from within.”
Slavov and his team use automation to identify, label, and categorize their analysis of cellular proteins to encourage supervised machine learning that can discern on its own what is significant.
“Fundamentally, any biological system is very complex, and science has had less success understanding complex systems,” says Slavov “With AI, the hope is that we can better understand the physical interactions between molecules, which can be broadly applicable to any disease and early human development.”
Slavov’s research has been funded by the Chan Zuckerberg Initiative, and he was recently named a prestigious Allen Distinguished Investigator and was awarded a $1.5 million three-year grant to further his novel research.
Better treatment of disease
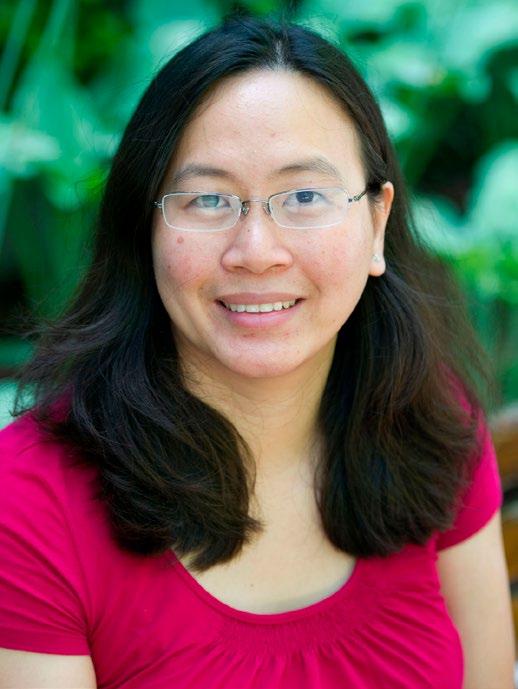
Jennifer Dy, professor, electrical and computer engineering
For more than a decade, Professor Jennifer Dy, electrical and computer engineering, has been working on a better way to identify and treat skin cancer. With her expertise in machine learning, Dy has been collaborating with optical engineer Dr. Milind Rajadhyaksha at the Memorial Sloan Kettering Cancer Center in New York, as well as a biomedical imaging colleague at Northeastern, Research Professor Dana Brooks of electrical and computer engineering.
The standard workflow for diagnosing skin cancer is dermoscopy using light, magnification, and the human eye, and often removing tissue for deeper inspection. Dy’s colleague in optical engineering is perfecting the use of reflectance confocal microscopy (RCM), which is newer equipment (and now a reimbursable procedure with a healthcare insurance billing code provided in 2017) that allows doctors to see lesions under the skin, minimizing the need for biopsy.
“While RCM is considered to be more effective and certainly less invasive, not many doctors are trained to use the equipment and analyze the 3D, grayscale images,” says Dy. “We’re applying AI and machine learning to automate where the dermal-epidermal junction is—which is usually where skin cancer starts and varies from person to person and from site to site—as well as detect patterns that signify cancerous areas.”
In another collaboration, Dy is partnering with experts in chronic obstructive pulmonary disease (COPD), which is a chronic lung function disease caused by smoking or the inhalation of pollution and other noxious particles. Doctors believe that COPD is heterogenous—meaning that people react differently to it based on unknown factors—and want to better stratify patients to offer them more effective treatments.
Together with Brigham & Women’s Hospital, Dy is a part of the COPDGene® Study, which is an NIH-funded investigation into possible genetic signifiers that effect disease progression. Researchers are collecting high-resolution computed tomography images, genetic data, and clinical data from a cohort of patients at 21 sites across the U.S., and it’s Dy’s group’s job to help use machine learning to understand COPD from this mountain of data.
“We’re looking at 10,000 patients with three different modalities over three separate time points and using AI to look for patterns that can help us discover COPD subtypes,” says Dy. “This is a rich problem from a modeling point of view in that there are thousands of possible relevant features in each modality, and we have to design methods to teach a computer how to decide which ones are important.”
While this particular study will clearly benefit the field of COPD disease research, Dy’s work on this difficult problem can help to advance machine learning as a field.
“While we are using machine learning to help make sense of the data we are giving it, we’re also focused on making it clearer how the computer arrives at its decisions,” explains Dy. “By showing doctors how the machine draws its conclusions, we’re able to also get their input and expertise on whether its methodology makes sense and helps them both understand and verify COPD’s characteristics.”
Improving a nationwide health crisis
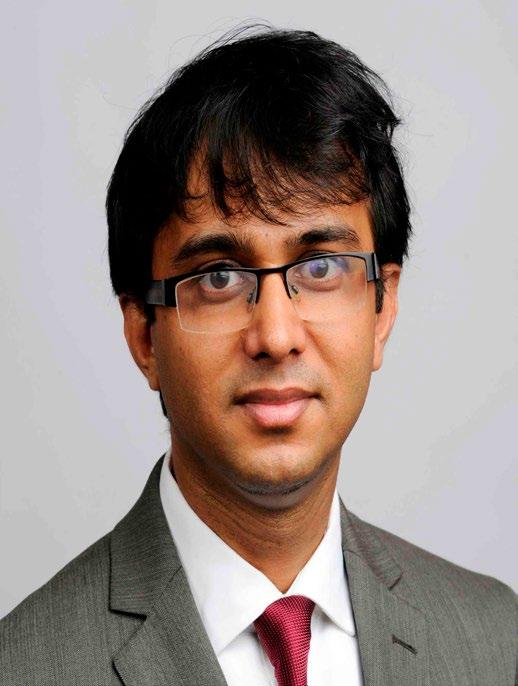
Md Noor E Alam, assistant professor, mechanical and industrial engineering
Assistant Professor Md Noor E Alam, mechanical and industrial engineering, is using machine learning techniques to analyze large-scale healthcare data and identity determinants that lead to opioid overdose.
Working from large-scale healthcare claim data from 0.6 million patients, the research team has identified 30 prominent features that may help indicate the possibility of opioid overdose in future patients. Many align with existing knowledge about opioid addiction behavior and some are brand new—and occasionally controversial— such as a history of certain mental illnesses and vaccinations.
Alam’s project is not only seeking to predict potential overdoses, but also taking a systems approach to see how they can help to address the nationwide issue on opioid addiction. Some of these projects are funded by the Centers for Disease Control in partnership with the Massachusetts Department of Public Health.
“Of the many people dying in the U.S. because of opioid overdose, more than 30% have been from prescribed medication,” says Alam. “So, our first step is to make sure that physicians are appropriately identifying patients who have the potential to misuse the drug.”
Because conventional machine learning algorithms can’t capture the clinical trajectories with co-occurring conditions of a patient’s health history, Alam is currently working with his student Md Mahmudul Hasan, PhD’21, industrial engineering, to develop deep learning technology to overcome this limitation.
“We’re planning to provide physicians with a visual tool to help them predict and better understand the current condition of their patients,” says Alam. “Once the deep learning is developed, we’re going to create an interface for them to evaluate the technology and help us finetune it to incorporate their important domain knowledge.”
Alam hopes that this research can not only help to lower the rate of opioid overdoses in the U.S., but also offer patients better treatment with less addictive drugs and prevent unwanted prescription opiates from entering the black market.
See Related Article: Making Machine Learning Safer for More Applications